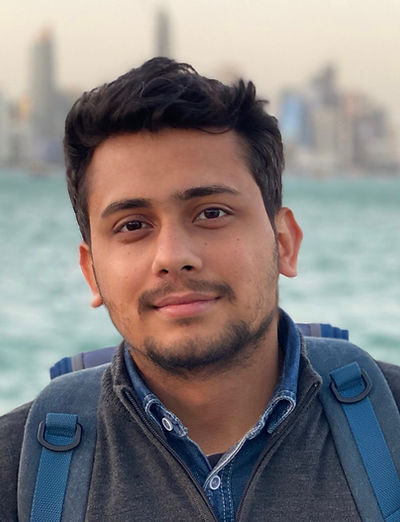
Proficient in:




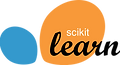
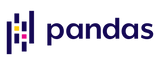

Kuntal Pal
Ph.D. Candidate in Physics
Myself, Kuntal. I completed my Bachelors and Masters in physics from IISER Kolkata. Currently, I'm a doctoral candidate in particle physics at University of California, Riverside.
My initial days of research as a graduate student involved mathematical modeling using quantum theory to explain different particle physics phenomena. For the past year and a half, I have been working on analyzing particle physics data from the Large Hadron Collider (LHC), which has gradually exposed me to the field of data science and machine learning. The journey since then has been nothing short of remarkable.
The initial introduction to big data and machine learning felt a little overwhelming but made me realize it was time to hit the basics. I decided to enroll in graduate-level courses from the computer science department. So far, Completed three graduate courses from the computer science department on Scientific Computing, Data Mining, and Optimization in Machine Learning and enrolled in one more on probabilistic models for Artificial Intelligence. These courses helped me gain experience through course projects by working on large datasets, implementing data mining, developing different deep learning models from scratch, and finally testing optimization algorithms suggested recently in the literature. Additionally, I have been taking relevant online courses to further diversify and update my expertise in this domain.
Apart from my deep interest in physics and machine learning, I'm very passionate about photography and love to capture nature at its best. I am fond of traveling and plan to travel and experience every corner of the world. On a not-so-warm evening, I am either sweating on the tennis court or biking around the neighborhood. I like to keep a positive attitude moving ahead in life and believe:
"Life is like riding a bicycle : To keep balance , you must keep moving - Albert Eienstein"
Data Science & ML Projects
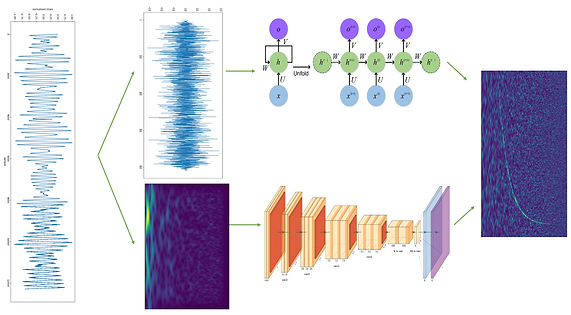
Performed time series classiffication for gravitational wave detection. Used RNN models after whitening and filtering of the raw data and also implemented different CNN models for time series data converted to spectrogram images using Q-Transform and Short-time Fourier transform. A total of five different neural network architectures are implemneted for classification.
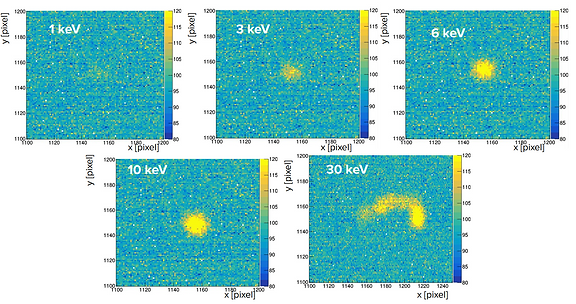
The task was to detect the presence/absence of neutral unknown (dark matter) particles by studying images of particle tracks from nuclear recoils while travelling through a gas mixture. Also the energy of the particles needs to be estimated. It was part of two separate kaggle competions during the 7th Summer school on Machine Learning in High Energy Phyics. Participated in both and finished 4th in one of them.
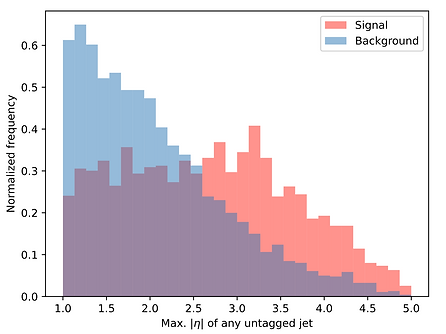
Devised feature engineering in collaboration with two others, using previous domain knowledge and data visualization to select 10 relevant variables as input to a classification model. Trained a boosted decision trees model with an ensemble of 800 trees as well as a deep neural network architecture to attain almost 93% accuracy in separting signal from the background.
Graduate CS courses at UCR
Online Courses
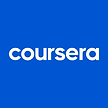
Machine Learning - Andrew Ng
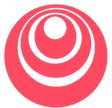
Deep Learning Specialization
-
Neural networks & Deep Learning
-
Improving deep neural networks.
-
Structuring Machine Learning Projects
-
Convolutional Neural Networks
-
Sequence Models
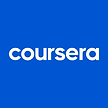
SQL for Data Science - UC Davis

Practical Data Science Specialization.
-
Analyze Datasets and Train ML Models
using AutoML.
Publications
​
-
Ultralight Thomas-Fermi dark matter. Kuntal Pal, Laura V. Sales and Jose Wudka (UC Riverside)